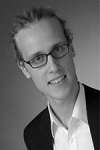
Oscar Koller
RWTH Aachen University, Germany
Title: How to Train a CNN on 1 Million Images When Your Data Is Continuous and Weakly Labelled: Towards Large Vocabulary Statistical Sign Language Recognition Systems
Biography
Biography: Oscar Koller
Abstract
Observing the nature inspires to find answers to difficult technical problems. Gesture recognition is a difficult problem and sign language is its natural source of inspiration. Sign languages, the natural languages of the Deaf, are as grammatically complete and rich as their spoken language counterparts. Science discovered sign languages a few decades ago and research promises new insights into many different fields from automatic language processing to action recognition and video processing. In this talk, we will present our recent advances in the field of automatic gesture and sign language recognition. As sign language conveys information through different articulators in parallel, we process it multi-modally. In addition to hand shape this includes hand orientation, hand position (with respect to the body and to each other), hand movement, the shoulders and the head (orientation, eye brows, eye gaze, mouth). Multi-modal streams occur partly synchronous, partly asynchronous. One of our major contributions is an approach to training statistical models that generalise across different individuals, while only having access to weakly annotated video data. We will focus on a new approach to learning a frame-based classifier on weakly labelled sequence data by embedding a CNN within an iterative EM algorithm. This allows the CNN to be trained on a vast number of example images when only loose sequence level information is available for the source videos. Although we demonstrate this in the context of sign language, the approach has wider application to any video recognition task where frame level labelling is not available.