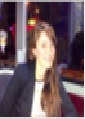
Biography
Biography: Cagcag Yolcu Ozge
Abstract
As a data mining field, analysis of time series has been one of the main research subjects for decades. In the literature, many models have been put forward for the prediction problems. Traditional prediction models, for modelling the time series, may fail to solve the prediction problems including complex real-world time series because of the several assumptions which need to be taken account of. These methods can be grouped as probabilistic methods. An effective way to predict time series has been to utilize advanced time series prediction models that can be grouped as non-probabilistic including fuzzy inference systems based on fuzzy sets and fuzzy arithmetic, and computational inference system based on artificial neural networks. Not requiring any assumption in prediction, therefore, makes advanced time series prediction models applicable for many fields. Multilayer perceptron (MLP) proposed by McCuloch and Pitts (1943) has been commonly used as a computational method. Single multiplicative neuron model (S-MNM) that does not contain this type of problem is introduced by Yadav et al. (2007). S-MNM uses a multiplicative function in its neuron as an aggregation function on the contrary to MLP that uses additive function. An ANN structure named linear and non-linear artificial neural network incorporating the properties of these two neural networks has been suggested by Yolcu et al. (2013). Fuzzy time series (FTS) approaches, introduced by Song and Chissom (1993), are another prediction tools that have been used efficiently in recent years. To improve prediction performance, Cagcag Yolcu (2013), and Cagcag Yolcu et al. (2016) put forward hybrid FTS models in which combined artificial neural networks and fuzzy clustering. In this talk, the reason of researchers need to use non-probabilistic prediction methods will be emphasized and some models in fuzzy inference systems and computational inference systems will be talked with their some applications.