Day 1 :
Keynote Forum
K Asari Vijayan
University of Dayton, USA
Keynote: Visual perception for autonomy application to object detection and behavior analysis in complex environmental conditions
Time : 09:15-10:00
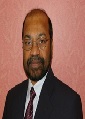
Biography:
Vijayan K Asari is a Professor in Electrical and Computer Engineering and Ohio Research Scholars Endowed Chair in Wide Area Surveillance at the University of Dayton (UD), Dayton, Ohio, USA. He is the Director of the Center of Excellence for Computer Vision and Wide Area Surveillance Research at UD. He received his PhD in Electrical Engineering from the Indian Institute of Technology, Madras in 1994. Prior to joining UD in February 2010, he worked as Professor in Electrical and Computer Engineering at Old Dominion University, Norfolk, Virginia for 10 years. He holds three patents and has published more than 600 research papers in the areas of image processing, pattern recognition, machine learning, neural networks and high performance embedded systems. He has supervised 26 PhD dissertations and 40 MS theses in electrical and computer engineering. Currently several Masters and Doctoral level graduate students are working with him. He has received several teaching, research, advising and technical leadership awards including the University of Dayton, School of Engineering Vision Award for Excellence in August 2017, the Sigma Xi George B Noland Award for Outstanding Research in April 2016, and the Outstanding Engineers and Scientists Award for Technical Leadership from The Affiliate Societies Council of Dayton in April 2015. He is a Senior Member of IEEE and SPIE, and is a co-organizer of several SPIE and IEEE conferences and workshops.
Abstract:
Automated visual monitoring involves data acquisition, analysis, and interpretation for understanding objects and object behaviors. Automated visual data analysis systems are mostly used for military, law enforcement and commercial applications. Sensors of different types and characteristics in various platforms are used for the acquisition of data. In recent years, there has been a spurt in the development of palm-sized cameras for the consumer market that are equipped with fish eye lenses on front and back sides to deliver 360 degree spherical views. Intelligent analysis of these data is an important task in applications such as automatic human detection, identification, activity recognition, behavior analysis, anomaly detection, alarming, etc. Object motion analysis and interpretation are integral components for activity monitoring and situational awareness. We present the development of a robust automated system which can detect and identify people using imagery captured using visible, infrared and omnidirectional cameras in a mobile platform and track their actions and activities by a spatiotemporal feature tracking mechanism. The automated visual analysis procedure includes preprocessing of data for distortion correction, novel methods for feature extraction, and machine learning based approaches for object classification.
Keynote Forum
Christl Lauterbach
Future-Shape GmbH, Germany
Keynote: SensFloor®: Human movement tracking and more
Time : 10:00-10:45
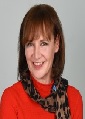
Biography:
Christl Lauterbach founded the Future-Shape GmbH, Germany in 2005 and is Managing Director since then. Main product of Future-Shape is SensFloor, a large-area sensor floor. From 1999 until 2005 she was at Infineon Technologies AG, Corporate Research, Senior Staff Engineer for Emerging Technologies, and Project Manager for Smart Textiles. Before that, she worked 22 years at Siemens AG, Corporate Research and Technology, as a developer for Semiconductor Technology and Circuit Design. Education: Assistance Degree in Electrical/Communication Engineering. She has more than 200 patents and patent pending, and >100 scientific publications
Abstract:
The described sensor floor is a textile-based large-area sensor system, which is installed as an underlay beneath the flooring. It detects people moving across the floor, calculates their trajectories and distinguishes between foot steps and a fall. The use of capacitive proximity sensing instead of pressure sensing gives high flexibility in floor design: even non-elastic flooring, like laminate parquet or tiles are suitable. The installed system is invisible and unobtrusive compared to camera systems. The SensFloor system enables a variety of different applications in the domain of ambient assisted living (AAL) like fall detection, activity monitoring, energy savings, control of automatic doors, intrusion alarm and access control. Presence detection and human movement tracking provide valuable data for Internet of things (IoT) scenarios like i.e. behavioral analysis in retail, workspace, living or healthcare. For example, it allows care organizations to optimize their workflows and improve quality of care. In future, artificial intelligence will provide means to evaluate the health status of people walking on the floor. The proposed processing scheme is able to extract behavioral information from the sensor data, which is fed into a learning algorithm that internally represents typical patterns, and outputs a measure for the divergence of current behavior from typical behavior. Gait pattern analysis by SensFloor provides objective data for medical diagnosis by medical experts like neurologists or physiotherapists. Gait parameters like walking speed, step length, and straightness are important factors for the health status of patients. Regular assessments during the treatment indicate the success of medication and rehabilitation.
Keynote Forum
Andreas Greilhuber
Chief Operating Officer Anyline.com, Austria.
Keynote: The journey from convolutional neural network to real customer value
Time : 11:05-11:50
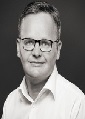
Biography:
Andreas Greilhuber is a Computer Scientist by Profession and maker of large-scale services business units (gained experience with IBM, PricewaterhouseCoopers - 20 years’ experience with Technology and Consulting Firms, managed large- scale services business units in Europe, Middle-East and Africa). Overlooking an information technology era from PC to Artificial Intelligence with an experience in a wide range of disruptive technologies and business models (e.g., early adoption of IBM Watson in German-Speaking Market in the Health Industry), he decided to leave corporates and make AI real with an Austrian start- up. He is currently engaged as Chief Operations Officer with ANYLINE, Business Developing and Advisor of an innovation platform Innovation 4.x. located in Linz, Tel Aviv and Belin.
Abstract:
Anyline.com is one of the most promising AI companies in Europe. Founded in 2013 – Anyline teaches smart phones reading. The start was a specific use-case and customer need to read the blood sugar devices for diabetes patients – a clear need and customer pain with a solution to bridge the analog and digital world with the smart phone camera. The solution was patented. We were struggling what to do next with our patented solution and decided to develop an offering for the utilities industry. Similar to the blood sugar devices there are more enough analog and digital electricity, water, heat and gas meters around the world. Even compelling events like the European smart meter roll-out existed, but where is the real customer value of a vertical integrated solution powered by AI and why B2B customers shall pay for. Step by step we evolved our business model from solving mobile app developer’s pain to use AI based technologies like TensorFlow to the right vertical integrates solutions for law enforcement, automotive, border control and finally developed a successful product and solution approach to generate the funds needed to further push the AI adoption together with our customers.
- Animation and Simulations | Artificial Intelligence | Image Processing | Computer Vision & Pattern Recognition | Multimedia Networking|Internet of Things
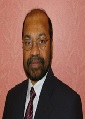
Chair
K Asari Vijayan
University of Dayton, USA
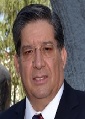
Co-Chair
Hector Perez-Meana
National Polytechnic Institute, Mexico
Session Introduction
Haipeng Peng
Beijing University of Posts and Telecommunications, China
Title: Semi-tensor product compressive sensing and its application in image processing
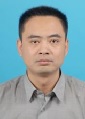
Biography:
Haipeng Peng received the MS degree in System Engineering from Shenyang University of Technology, Shenyang, China, in 2006, and the PhD degree in Signal and Information Processing from Beijing University of Posts and Telecommunications, Beijing, China, in 2010. He is currently a Professor at the School of Cyber Space Security, Beijing University of Posts and Telecommunications, China. His research interests include information security, network security, complex networks and control of dynamical systems. He is the co-author of over 100 scientific papers. His SCI citations of other scholars are over 1400 times, and his Google citations are over 2700 times.
Abstract:
Compressive sensing (CS) is a popular research at home and abroad. One of the key bases of modern signal processing is the Shannon-Nyquist sampling theory, where the number of discrete samples required for a signal that can be reconstructed without distortion is determined by its bandwidth. As a new sampling theory, CS can obtain discrete samples of the signal by random sampling, which is far less than the Shannon-Nyquist sampling rate by the sparse characteristic of the signal, and then CS can reconstruct the original signal by non-linear reconstruction algorithms. CS was proposed by Terence Tao (the winner of Fields Medal), Emmanuel Candès (IEEE Fellow), and David Donoho (the member of the US National Academy of Sciences) in 2004. This theory has received wild attention in academia and industry once it has been put forward. It has been applied into the fields of signal processing, digital communication, network security, image processing, medical imaging, geologic survey, radiating systems etc. It has been rated as the top 10 scientific and technological progress in 2007 by American Science and Technology Review. This speech focuses on the latest research progress of semi-tensor compressive sensing theory and its application in image processing. This speech introduces the basic theory of CS from the point of view of collecting data more effectively, and introduces our newly proposed semi-tensor compressive sensing theory, which breaks through the limitation of traditional compression perception matrix. The dimension of the measurement matrix that needs to be processed during the sampling recovery process is greatly reduced so that the signal is processed and restored at a lower sampling rate than the classical CS theory.
Qaysar Salih Mahdi
Ishik University, Iraq
Title: Evaluation of mobile GSM performance under different atmospheric propagation models
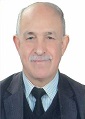
Biography:
Qaysar S Mahdi has completed his PhD from Engineering Military College and Postdoctoral studies from Engineering Military College. He is the Director of IT Services & Website Department; Ishik University. He has published more than 15 papers in reputed journals and has been serving as an Editorial Board Member of repute.
Abstract:
Atmospheric propagation is very effective on the performance of the wireless, mobile, radar, and communication systems. In this paper different atmospheric models are constructed under different atmospheric conditions. The performance of a GSM mobile communication is tested under different atmospheric models. From the obtained results, it is noticed that the coverage of the mobile system antenna is changed highly if the refractive index model of certain country is changed. It is concluded that the atmospheric propagation is very essential parameter to be taken into account when the siting of a mobile GSM network is to be evaluated and designed. This study will be very useful in order to predict the performance of ground radio and airborne systems.
Byung Geun Lee
Gwangju Institute of Science and Technology, South Korea
Title: Recent research on hardware neural network
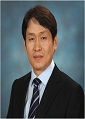
Biography:
Byung Geun Lee received the BS degree in Electrical Engineering from Korea University, Seoul, South Korea, in 2000; the MS and PhD degrees in Electrical and Computer Engineering from the University of Texas at Austin, in 2004 and 2007, respectively. From 2008 to 2010, he was a Senior Design Engineer at Qualcomm Inc., San Diego, CA, USA, where he had been involved in the development of mixed-signal ICs. Since 2010, he has been with the Gwangju Institute of Science and Technology. He is currently an Associate Professor with the School of Electrical and Computer Science. His research interests include high-speed data converter, CMOS image sensor, and neuromorphic system developments.
Abstract:
An artificial neural network (ANN) is a computational model inspired by neo-cortex of human brain that is capable of solving a variety of problems in recognition, prediction, optimization, and control. It can be also described as a network of synaptically connected neurons that can create, modify, and preserve information through sequential learning procedures. Recently, hardware implementation of artificial neural network called hardware neural network (HNN) is gaining popularity due to its potential usability for industrial applications requiring recognition, optimization, and prediction using complex data sets. However, hardware implementing issues need to be solved for the widespread of HNN. In this presentation, I will summarize recent efforts of HNN implementations with pros and cons of each approach.
Lixiang Li
Beijing University of Posts and Telecommunications, China
Title: Foraging behavior of ants and its application in optimization field
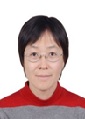
Biography:
Lixiang Li is currently a Professor at the School of Cyber Space Security, Beijing University of Posts and Telecommunications, China. She received the PhD degree in Signal and Information Processing from Beijing University of Posts and Telecommunications, Beijing, China, in 2006. Her interests include compressive sensing, swarm intelligence, neural networks and complex networks. She is the co-author of more than 150 papers. Her SCI citations of other scholars are over 2000 times, and her Google citations are over 3400 times. In 2014, her result of swarm intelligence published in PNAS has been widely reported by more than 200 domestic and foreign media (such as Time Magazine, Science Daily, the Christian Science Monitor, the Daily Mail, Science and Technology Daily and etc.). In 2015, her result of memory resistance neural network published in EPJB was assessed as highlight paper and was reported by at least 28 international media.
Abstract:
Ants and other social animals have captured the attention of many scientists because of their self-organizing behavior and the high level of structuration their colonies can achieve, especially when compared to the relative simplicity of the individuals. The study of the foraging behavior of group animals (especially ants) is of practical ecological importance, but it also contributes to the development of widely applicable optimization problem-solving techniques. In recent years, algorithms inspired by models of animal group behaviors have achieved increasing success among researchers in computer science, communication networks and operations research. This talk introduces basic mechanisms of effective foraging for social insects or group animals that have a home. The whole foraging process of ants is controlled by three successive strategies: hunting, homing, and path building. These learning strategies have advantages on the internet optimization process. This speech also introduces some dynamical models of ant foraging. We introduce the influences of the special region around the nest, the size of the food source, the search range, the limitation of ants’ physical ability, and ants’ learning process with respect to foraging behavior. Our analysis suggests that group animals that have a home do not perform random walks, but rather deterministic walks in a random environment. They use their knowledge to guide them and their behavior is also influenced by their physical abilities, their age, and the existence of homes. In this talk, we will also introduce the application fields of ant foraging behavior, such as network optimization, signal processing, network security, distributed control et al.
Hanmin Jung
Korea Institute of Science and Technology Information, South Korea
Title: Detectionof uneven road surfaces on internet of vehicles
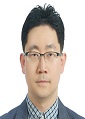
Biography:
Hanmin Jung works as the Head of Scientific Data Research Center and Chief Researcher at Korea Institute of Science and Technology Information, Korea since 2004. He received his BS, MS, and PhD degrees in Computer Science and Engineering from Pohang University of Science and Technology, Korea in 1992, 1994, and 2003. Previously, he was Senior Researcher at Electronics and Telecommunications Research Institute, Korea, and worked as CTO at DiQuest Inc., Korea. Now, he is also full Professor at University of Science & Technology, Korea; Visiting Professor at National Human Resources Development Institute, Korea; Visiting Fellow at University of Southampton, UK; Guest Professor at Graz University, Austria; Guest Professor at Paderborn University, Germany; Editor at Korea Contents Association; Director at Korean Society for Internet Information; Director at Korean Society for Big Data Service; Director at the Korean Society of Computer and Information and; Committee Member of ISO/IEC JTC1/SC32. His current research interests include the fourth industrial revolution, semantic web, artificial intelligence, text mining, big data, information retrieval, human-computer interaction (HCI), data analytics, natural language processing (NLP), and Internet of Things (IoT).
Abstract:
This study aims at finding uneven road surfaces on an Internet of Vehicles (IoV) network composed of about 40 taxis and 2 dedicated vehicles. The taxis are operating in Daegu city, which is the 4th largest metropolitan city of 883.56 km2 in Korea, for 7 months and are transmitting sensor data every 10 seconds. Thus, about 1.1 million sensing points per month are generated from the network. There are 10 types of sensors including vibration sensor, particulate matter sensors (PM10 and PM2.5), carbon monoxide (CO) sensor, and nitrogen dioxide (NO2) sensor inside taxi cab light. For the dedicated vehicles, 32 types of sensors including vibration sensor, acceleration sensor, gyro sensor, and black box are mounted inside hard-shell carrier. In this study, we’ve drawn a heat map as shown in fig. 2 with the vibration-related sensor data without pre-fixed road segmentation. Intensive road imbalances have been observed in major arterial roads leading from Daegu city hall (center in the map) to Seongseo industrial complex (left in the map), which imply that such roads are affected by frequent traffic and heavy trucks. Field inspections allowed us to identify a series of portholes and cracks at these points, many of which occur on maintained roads. It indicates that it is necessary to consider maintenance method for preventing and repairing potholes and pavement cracks as well as various environmental factors such as temperature, humidity, and traffic volume. Future study will include the monitoring and analysis of road conditions for other cities in Korea and abroad, and tracking the changes of the conditions through stable IoV deployment. Further, we will investigate the relationships among the factors affecting road imbalances on major bridges as such abnormality causes serious disaster.
Michael Robert Doran
Translational Research Institute - Queensland University of Technology, Australia
Title: Use of multimedia in grant applications
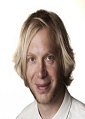
Biography:
He then completed a combined Postdoctoral Fellowship at the University of Queensland and Mater Medical Research Institute. He is a National Health and Medical Research Fellow and an Associate Professor at the Queensland University of Technology (Australia). Currently his laboratory is located at the Translational Research Institute (TRI) on the Princess Alexandra Hospital campus in Brisbane. His group’s multidisciplinary research interests include the study of bone, bone marrow, cartilage, and cancers that metastasize to the bone.
Abstract:
The Problem: Researchers and funding organizations are struggling with the ever increasing time and effort needed to prepare and review grant proposals. Ioannidis argued in Nature that burdensome funding systems mean that “scientists don’t have time for science any more”. We estimated that Australian researchers invest an average of 38 days preparing each new NHMRC Project Grant proposal, and 28 days on a resubmission. Based on anecdotal data and the similarity of the systems, we believe that ARC Discovery Project time burdens are similar. In 2017, Australian researchers submitted 3,136 ARC Discovery Project Grant proposals and 3,345 NHMRC Project Grant proposals. Assuming a conservative time of 28 working days per proposal, Australian researchers would have invested around 500 years preparing these proposals in 2017. Time is also needed to review proposals and in 2011 we estimated that $1,700 dollars of reviewer time is needed to review a project grant proposal, giving an estimated $5.6 million in review costs for NHMRC Project Grants alone. Despite the enormous investment by applicants and reviewers, estimates are that for one-third of grant proposals, success is somewhat random because of the variability among peer reviewers.
Necessary Change: The grant preparation and review systems must improve to address the current challenges. Funding allocation should remain merit-based, but preparing proposals should be less burdensome. Written proposals are often dense and tiring to review. The proposal format should engage reviewers and clearly contain the detailed information needed to assess the proposal’s feasibility, novelty and impact.
Possible Solution: Recently we argued in Nature, Trends in Biochemical Sciences, a Cell Press Video and Nature Index that an effective mechanism to enhance communication between applicants and reviewers was through video. Researchers routinely prepare PowerPoint presentations for conferences and record such presentations as lecture material. PowerPoint presentations with voice recordings are a logical potential alternative to written project descriptions. Such videos may be highly effective at transferring the key ideas from the minds of the authors to the reviewers, leading to better decision-making. This talk will outline our preliminary data and discuss the merits of trailing 15-minute PowerPoint presentations, with voice recording, as an alternative to traditional text-based grant applications. We reason that this approach will enable more effective and efficient communication and more reliable ranking of proposals than current written grant project descriptions.
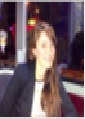
Biography:
Cagcag Yolcu Ozge is a Professor at Department of Industrial Engineering of Giresun University, Turkey. She received the MSc (Thesis title: Optimization of patient waiting time in the department of brain surgery of Ondokuz Mayis University by simulation) and the PhD (Thesis title: A new hybrid fuzzy time series approach) degrees in statistics at the Faculty of Science and Arts of the University of Ondokuz Mayis, Turkey, in 2010 and 2013, respectively. She was situated in the Robotics Research Group, Department of Informatics at King’s College London for a year in 2015 for Postdoctoral research. She has her expertise in time series analysis, fuzzy inference systems, artificial neural networks, artificial intelligence optimization algorithms, and robust statistics. She has various studies on time series prediction models including fuzzy inference systems and computational systems.
Abstract:
As a data mining field, analysis of time series has been one of the main research subjects for decades. In the literature, many models have been put forward for the prediction problems. Traditional prediction models, for modelling the time series, may fail to solve the prediction problems including complex real-world time series because of the several assumptions which need to be taken account of. These methods can be grouped as probabilistic methods. An effective way to predict time series has been to utilize advanced time series prediction models that can be grouped as non-probabilistic including fuzzy inference systems based on fuzzy sets and fuzzy arithmetic, and computational inference system based on artificial neural networks. Not requiring any assumption in prediction, therefore, makes advanced time series prediction models applicable for many fields. Multilayer perceptron (MLP) proposed by McCuloch and Pitts (1943) has been commonly used as a computational method. Single multiplicative neuron model (S-MNM) that does not contain this type of problem is introduced by Yadav et al. (2007). S-MNM uses a multiplicative function in its neuron as an aggregation function on the contrary to MLP that uses additive function. An ANN structure named linear and non-linear artificial neural network incorporating the properties of these two neural networks has been suggested by Yolcu et al. (2013). Fuzzy time series (FTS) approaches, introduced by Song and Chissom (1993), are another prediction tools that have been used efficiently in recent years. To improve prediction performance, Cagcag Yolcu (2013), and Cagcag Yolcu et al. (2016) put forward hybrid FTS models in which combined artificial neural networks and fuzzy clustering. In this talk, the reason of researchers need to use non-probabilistic prediction methods will be emphasized and some models in fuzzy inference systems and computational inference systems will be talked with their some applications.
Tian Tian
China University of Geosciences, China
Title: A biologically inspired spatio-chromatic feature for color object recognition
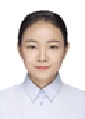
Biography:
Tian Tian received her BS and PhD from Huazhong University of Science and Technology (HUST), China, and visited Oakland University as a Visiting Scholar in 2012. She is currently with School of Computer Science, China University of Geoscience, Wuhan, China. Her major interests include computer vision and remote sensing image processing, and she has worked on feature extraction and image classification methods since the research of postgraduate period. Currently, she focuses on local image descriptors, biologically inspired image processing and deep learning methods on remote sensing image classification.
Abstract:
Statement of the Problem: Color information has been acknowledged for its important role in object recognition and scene classification. How to describe the color characteristics and extract combined spatial and chromatic feature is a challenging task in computer vision. To deal with the description of colors, plenty of approaches have been proposed. To study our visual system and mimic its structure and mechanism has naturally been proposed in the research of perceiving colors.
Methodology: The color information processing is implemented under a biologically inspired hierarchical framework, where cone cells, single-opponent and double-opponent cells are simulated respectively to mimic the color perception of primate visual system. More detailed, so responses are obtained by linear combination of retinal cone-like responses generated by Gaussian functions. The receptive field of a do cell is seen as the overlap of two oriented cells with inverse phases, and the double-opponent channels are simulated with oriented filters of inverse phases. Then the robust SIFT feature is extended as a shape description on the processed opponent color channels to obtain a spatio-chromatic descriptor for color object recognition.
Findings: The biologically inspired method is tested for color object recognition task on two public datasets, and the results support the potential of the proposed approach.