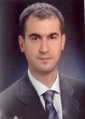
Aykut Koc
ASELSAN Research Center, Turkey
Title: Pattern recognition for architecture: Identifying interior styles and scene components from images
Biography
Biography: Aykut Koc
Abstract
The vast amount of user uploaded visual content available online makes automated visual classification a critical research problem. While existing studies for visual classification mainly focus on recognition of generic objects such as vehicles, plants, food and animals, recently, studies have also been presented for exploring a more challenging research problem, fine grained object classification, aiming to distinguish fine subcategories within coarse object categories, such as types of vehicles, flowers and kinds of food. Here, another fine grained categorization problem important for multimedia applications, categorizing in-building scenes and their architectural styles, is attempted which will be beneficial for applications related to real estate and interior decoration. In-building scenes are divided into five coarse categories; kitchen, bathroom, living room, bedroom and dining room. As fine categories, each in-building scene has been assigned an architectural style such as Asian, Contemporary, Victorian, Rustic and Scandinavian. On a database consisting of a large number of in-building images, descriptive patterns corresponding to types of scenes and specific architectural styles are learned globally by utilizing deep convolutional neural network based models that have proven success in visual categorization. Moreover, local scene elements and objects which provide further clues for identifying architectural styles are discovered: Scene objects with unique architectural style characteristics carry more discriminative power, whereas co-existing objects visible among various types of scenes are less discriminative. As potential useful applications, several scenarios for classification and retrieval of in-building images are investigated. Experiments show that using only the learned deep representations are effective in identifying scene types while they perform poorly for architectural styles. Nonetheless, revealing key local scene objects ameliorates their performance for both classification and retrieval tasks for architectural styles.