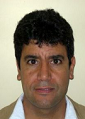
Mounîm A. El-Yacoubi
University of Paris-Saclay, Palaiseau, France
Title: Sequential modeling and recognition of human gestures and actions
Biography
Biography: Mounîm A. El-Yacoubi
Abstract
Human action recognition (HAR) is an active research field, driven by the dramatic price decrease of powerful digital cameras, storage and computing machines, and by the potential of HAR for designing smart engines making sense of today ubiquitous video streams. In video surveillance, for instance, HAR can help determining abnormal events in public facilities. For e-health, HAR may be harnessed as an assistive technology monitoring people with autonomy loss. Recognizing human actions, however, is challenging. Known variability factors such intra-class and inter-class variability, are much more adverse as they involve additional structural variability that is hard to cope with. Besides, actions are not communicative in general, which hinders relevant information acquisition and tracking. Others variability sources include viewing direction and distance w.r.t acquisition sensor, lighting conditions, etc. In this talk, we review the problem of human action and gesture recognition in general. After discussing the challenges above, we propose a new approach that focuses on video sequential input modeling. The modeling is based on a two-layer SVM – Hidden Conditional Random Field (SVM-HCRF) in which SVM acts as a discriminative front-end feature extractor. First, a sliding window technique segments the video sequence into short overlapping segments, described each by a local Bag-of-Words (BOW) of interest points. A first-layer SVM classifier converts each BOW into a vector of class conditional probabilities. The sequence of these vectors serves as the input observation sequence to HCRF for actual human action recognition. We show how this hierarchical modeling optimally combines two different sources of information characterizing motion actions: local motion semantics inferred by SVM, and long range motion feature dependencies modeled by HCRFs at a higher level. Finally, we show how these models can be extended to the problem of conjoint segmentation and recognition of a sequence of actions within a continuous video stream.